Data has become one of the most valuable assets for organizations seeking to innovate and stay competitive. Leveraging data through AI and machine learning can lead to breakthrough insights and smarter decision-making. However, the path to achieving these insights is often obstructed by challenges in data integration. Integrating diverse data sources into a unified framework is essential for effective AI applications, but it can be a complex and time-consuming process.
MongoDB simplifies data integration with its flexible schema design, enabling organizations to easily manage and unify different types of data. Additionally, its Atlas platform provides powerful tools for seamless data access and analysis, helping businesses leverage AI to gain faster, deeper insights and make better decisions.
The challenge: successful data integration for AI initiatives
Understanding the complexity
Data integration poses significant challenges for organizations, especially when data is spread across different departments and stored in varied formats. This scenario, known as data silos, creates barriers to achieving a comprehensive view of the organization’s information landscape. Different data types – structured, unstructured, text, images – each require unique processing techniques, which complicates integration efforts.
Additionally, the need for real-time data processing adds another layer of complexity. As businesses aim to respond more quickly to market changes and customer demands, they require systems capable of handling continuous data streams efficiently. However, integrating these diverse data sets in real-time can be a daunting task, often leading to delays and increased costs.
Impact on AI and ML models
Integration issues directly affect the performance and accuracy of AI and ML models. These models depend on well-organized, clean data to make accurate predictions and provide valuable insights. When data is fragmented or inaccessible, the quality of AI outputs can suffer, potentially leading to incorrect conclusions and suboptimal business decisions. Models might overlook important patterns or fail to recognize critical trends due to incomplete or inconsistent data inputs.
Furthermore, data scientists often find themselves spending more time on data preparation and cleaning than on model development and analysis. This not only slows down the pace of AI projects but also limits the ability of businesses to quickly adapt to new information and evolving market conditions. Inefficient data integration can thus stifle innovation, reducing an organization’s ability to capitalize on new opportunities.
What we need is a unified approach
Addressing these challenges requires a unified data strategy that breaks down silos and facilitates seamless integration across all data sources. A cohesive data platform allows organizations to streamline the flow of information, making it more accessible and ready for analysis. By minimizing the time and effort needed for data preparation, a unified approach can significantly enhance the speed and agility of AI-driven initiatives.
Such a strategy also supports better data governance and compliance, ensuring that data is not only integrated but also secure and properly managed. This holistic approach to data management is essential for deriving accurate, timely insights that drive informed decision-making and foster innovation.
MongoDB to the rescue: enhancing data integration with flexible schema design and Atlas vector search
Flexible schema design for dynamic data environments
A dynamic data environment requires a flexible approach to schema design, and MongoDB excels in this regard. Unlike traditional databases that rely on rigid, predefined schemas, MongoDB’s flexible schema design allows organizations to adapt quickly to changing data requirements. This adaptability is particularly valuable for enterprises dealing with a wide variety of data types and structures.
Adaptability and scalability
With MongoDB, businesses can integrate diverse datasets without the need for extensive schema migrations, which can be time-consuming and costly. This capability is especially important in AI initiatives, where data types and sources frequently evolve. MongoDB’s flexible schema accommodates these changes seamlessly, enabling organizations to scale their data operations efficiently and without disruption.
Reduced time to insight
The flexibility of MongoDB’s schema design also translates into a reduced time to insight. Traditional data preparation processes can be cumbersome, often requiring extensive data cleaning and transformation to fit into rigid schemas. In contrast, MongoDB’s approach simplifies these steps, allowing data teams to spend more time on analysis and less on preparation. This not only speeds up the development of AI models but also ensures that insights are derived more quickly, allowing businesses to respond faster to market dynamics and customer needs.
Harnessing the power of Atlas vector search for AI-driven insights
MongoDB’s Atlas Vector Search enhances data integration efforts by enabling sophisticated search capabilities across vast datasets. This feature is particularly beneficial for AI applications that rely on recognizing patterns and performing similarity searches.
Enhanced data retrieval
Atlas Vector Search allows businesses to efficiently retrieve relevant data from large, complex datasets. This is crucial in AI scenarios where the ability to quickly find and analyze similar data points can significantly impact the performance of models, such as in recommendation systems or anomaly detection. By streamlining the data retrieval process, Atlas Vector Search helps ensure that AI models have access to the most pertinent data, thereby enhancing their accuracy and reliability.
Improving AI model accuracy
By integrating data from multiple sources using vector search, organizations can achieve a deeper contextual understanding of their datasets. This holistic view enables AI models to make more nuanced and accurate predictions. For instance, in customer segmentation or fraud detection, combining structured and unstructured data through vector search provides a richer, more detailed perspective. This capability not only improves model accuracy but also supports faster decision-making, helping businesses stay competitive by responding quickly to emerging trends and challenges.
Business value and competitive advantage
The ability to integrate data more effectively and retrieve insights quickly provides a significant business advantage. Efficient data integration drives innovation by allowing organizations to experiment with new AI models and approaches more freely. It also enhances customer experiences by enabling more personalized and responsive interactions. By staying ahead of the curve in data integration and AI, businesses can uncover new growth opportunities, streamline operations, and maintain a competitive edge in their industry.
Summary
Data integration remains a significant hurdle for businesses aiming to leverage AI for strategic advantage. Overcoming this challenge requires a thoughtful approach that combines flexible data management with powerful analytical tools. MongoDB offers organizations a compelling solution to these challenges with schema design and Atlas vector search. These can be highly efficient when used with the right set of tools, which enable organizations to easily navigate and understand their schema, make it simpler for them to manage diverse data types as well as streamline the integration process by having a clear view of the structure/organization of the data. Effective and precise querying and aggregation building can also help ensure datasets for AI are accurate.
By addressing the complexities of data integration, organizations can ensure that their AI initiatives are not only effective but also agile and responsive to changing needs. Leveraging these capabilities and tools enables businesses to drive innovation, enhance customer experiences, and make data-driven decisions with greater confidence. As the market landscape continues to evolve, adopting technologies and tools that simplify data integration and enhance AI capabilities will be crucial for staying competitive and achieving long-term success.
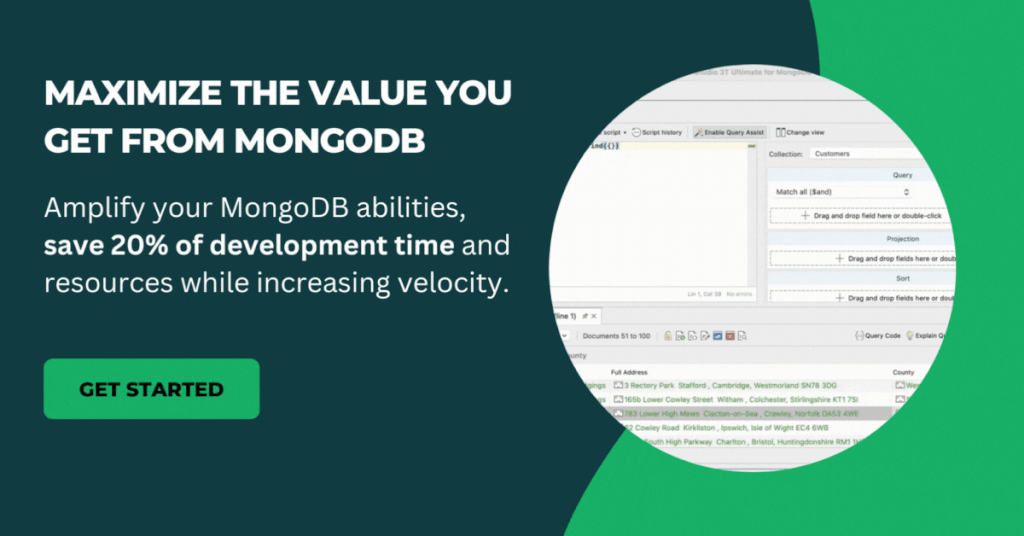