Time series data is data gathered over time, like sensor readings or stock price history. MongoDB Time Series collections allow the repetitive records of time series data to be stored and queried efficiently in a MongoDB database.
Studio 3T added support for Time Series collections in version 2022.9.
In this article, we’ll explain what a Time Series is in general and how MongoDB Time Series collections make it more efficient to store and query those collections.
What is a Time Series
Time Series is a term for data where the most important field is the timestamp, because each entry in a time series represents data for that time.
So say you have a thermometer, it records temperature every minute:
10:00,25.1
10:01,25.2
10:02,25.1
10:03,24.7
This isn’t our only thermometer, so we’d better identify it with some data about the data, the meta data:
Living Room,Sensor 1,10:00,25.1
Living Room,Sensor 1,10:01,25.2
Living Room,Sensor 1,10:02,25.1
Living Room,Sensor 1,10:03,24.7
Now, to store this data in MongoDB we’ll turn it into JSON. (And make those times into proper ISODate types):
{
timestamp: ISODate("2022-10-06T10:00:00.000Z"),
location: {
room: "Living Room",
sensor: "Sensor 1"
},
temperature: 25.1
}
{
timestamp: ISODate("2022-10-06T10:01:00.000Z"),
location: {
room: "Living Room",
sensor: "Sensor 1"
},
temperature: 25.2
}
{
timestamp: ISODate("2022-10-06T10:02:00.000Z"),
location: {
room: "Living Room",
sensor: "Sensor 1"
},
temperature: 25.1
}
{
timestamp: ISODate("2022-10-06T10:03:00.000Z"),
location: {
room: "Living Room",
sensor: "Sensor 1"
},
temperature: 24.7
}
This is easy to import into MongoDB, but consider the following things:
- To average the temperature for those four minutes would involve retrieving and aggregating four different documents.
- Each one of those four documents repeats the same values for the location and sensor information.
Doesn’t sound too bad does it? Until you think of, for example, measuring the temperature every second, for a hundred rooms, each with ten sensors in them.
Doing calculations over time involves searching hundreds of documents in MongoDB. Indexing the location and sensor and time gives you some big indexes, so that’s not an option. Also there’s going to be a massive amount of repetition in the database, chewing up all your storage.
Compressing the data
What we’d ideally like to do, in order to save space, is accumulate all the entries for one particular location in a single record.
{
location: {
room: "Living Room",
sensor: "Sensor 1"
},
Data: {
timestamp: {
0: ISODate("2022-10-06T10:00:00.000Z"),
1: ISODate("2022-10-06T10:01:00.000Z"),
2: ISODate("2022-10-06T10:02:00.000Z"),
3: ISODate("2022-10-06T10:03:00.000Z")
},
temperature: {
0: 25.1,
1: 25.2,
2: 25.1,
3: 24.7
}
}
}
Now, to perform calculations, we only have one document to retrieve and the metadata only appears once. Each field’s data point is also indexed so that it should be even quicker to retrieve.
But this is also a huge pain to manage. And MongoDB documents max size is 16MB so we can’t keep everything in one document. We could divide them up into blocks of time with a document per block, but oh no! Then it’s even more complicated!
And that’s where MongoDB Time Series collections comes to the rescue.
What are MongoDB Time Series Collections?
What if we could tell MongoDB that one field is a timestamp and another is metadata and everything else was data points? And what if MongoDB did all the transformational magic for you to make things quicker and more compressed? And what if you could carry on working with a collection, as simple, uncompressed documents and have MongoDB speed up all your operations?
That’s what a MongoDB Time Series collection does. You create a MongoDB Time Series collection in Studio 3T like any other collection, but at creation time you can tell MongoDB the name of the field with the timestamp in it and the name of the field with the meta data. Let’s create a roomtemps
collection.
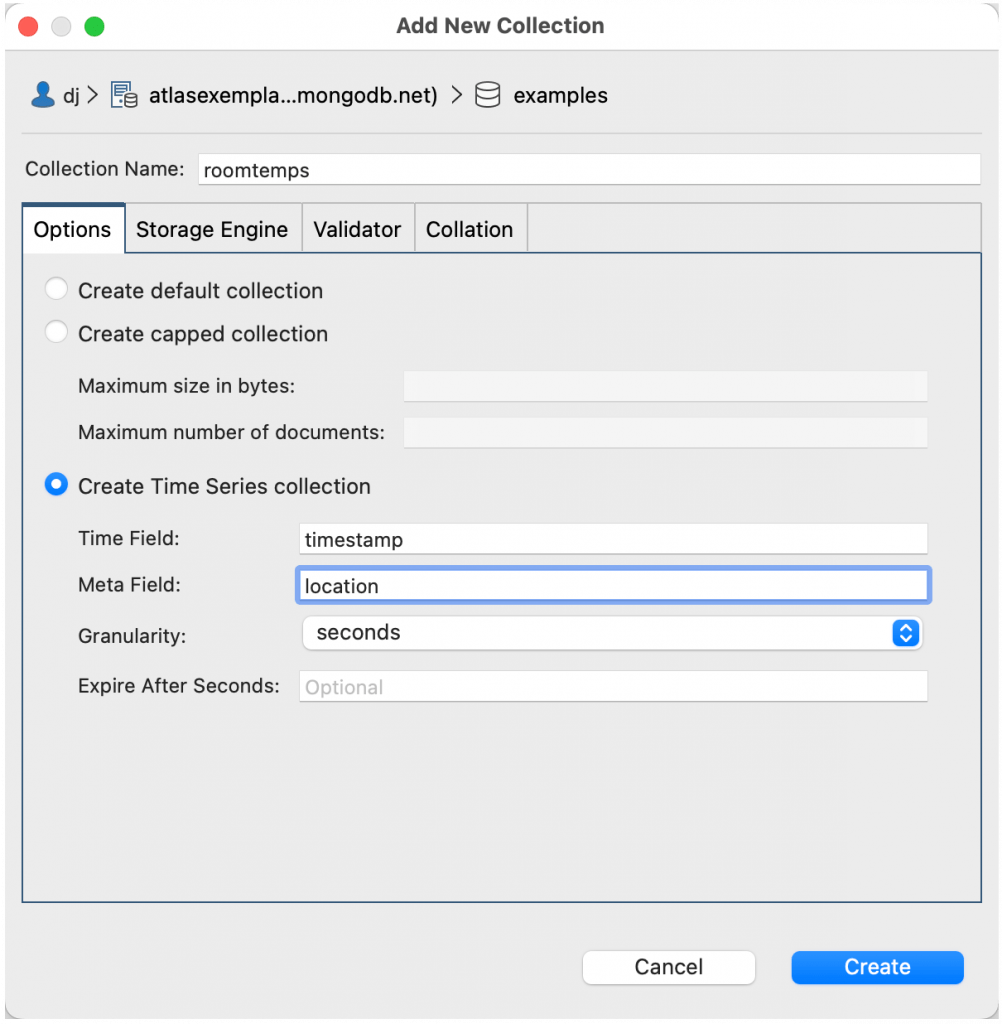
Our time field is timestamp
and our meta field is location
.
The granularity setting is a hint to MongoDB as to what to expect in terms of density of data points. It defaults to seconds, but if your data comes in every minute or hour, you may want to adjust it. The Expire After Seconds is also optional. Setting this can delete your data in a time series collection after a certain amount of time. That’s useful if your collection is only of “hot” or recent data. We’ll leave both of those alone, and click on create.
We can now add our JSON documents from earlier, exactly as they are shown and then query the collection:
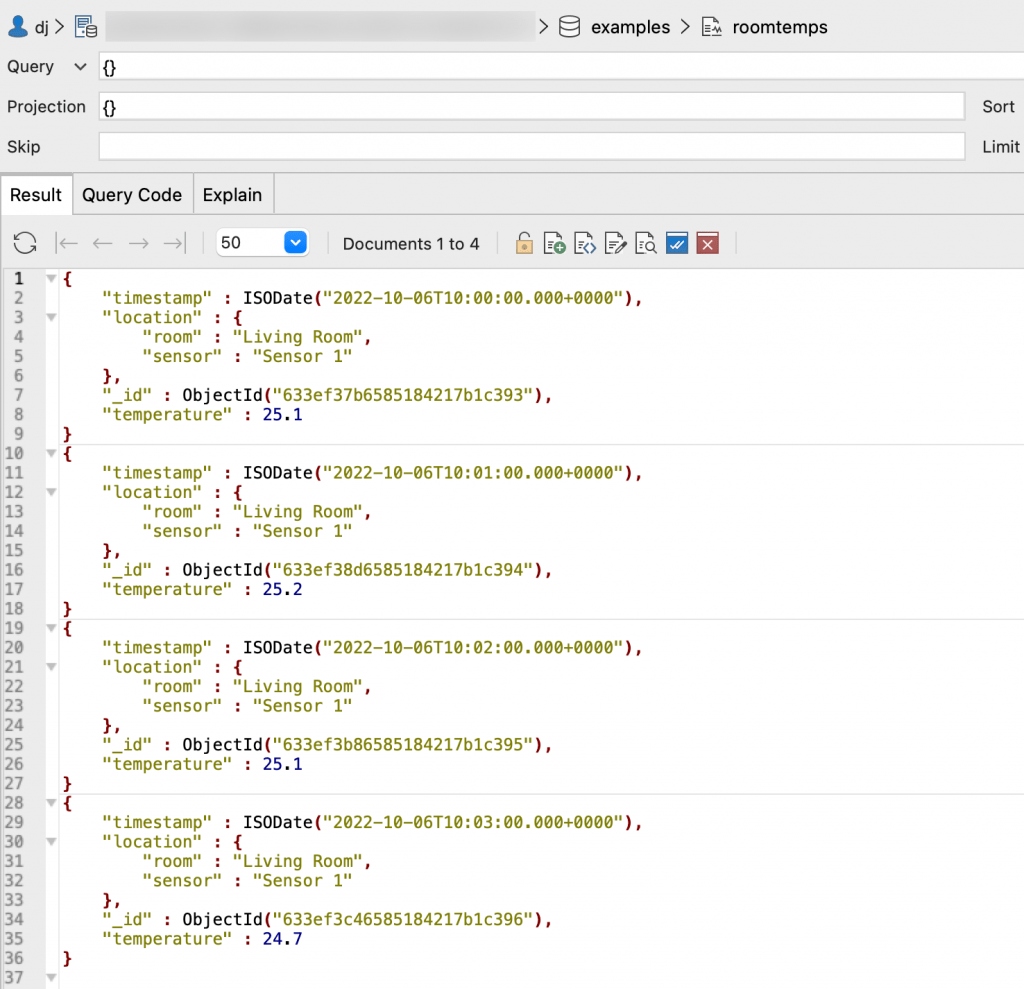
This looks pretty much the same as the documents we just inserted. That’s because in the background, MongoDB created a system collection – system.buckets.roomtemps
– to store the data in, in a format that looks more like our single-document-with-all-the-data from earlier.
The collection we are working with – roomtemps
– looks like a simple collection of single, point-in-time documents, because that’s how we want to visualize our Time Series data in MongoDB, so it’s easy to work with in aggregation.
If we look at system.buckets.roomtemps collection we can see our compressed document.
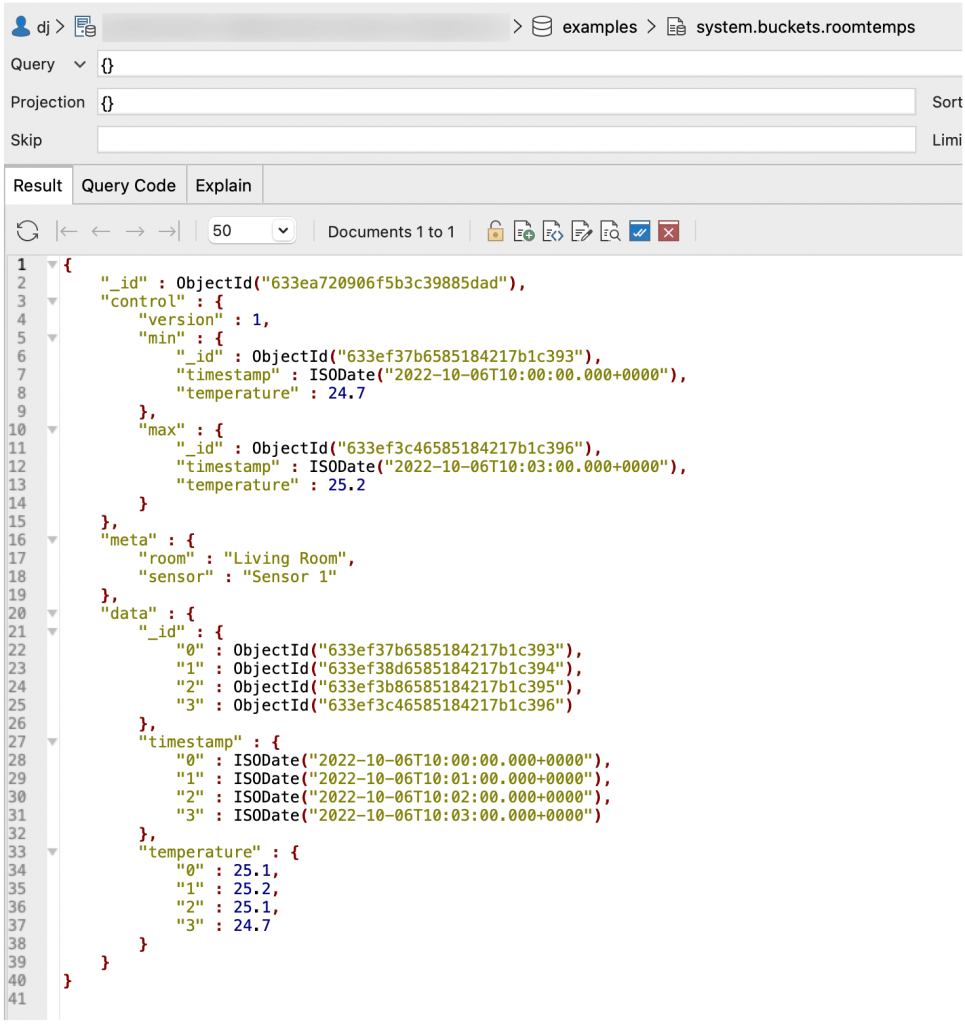
There’s one difference – there’s a control section which holds minimum and maximum values for all the data fields. Why? Optimization! You don’t need to do anything when querying this data but MongoDB can use the min and max values to quickly find bits of the time series or values which are within ranges. And because many time series queries are “What happened over this range of time”, it really can optimize the queries.
Indexes and Time Series Collections.
With all this optimization, there’s still one thing that can be done to boost query performance on Time Series collections. Adding a secondary index on the metadata’s fields (and adding the timestamp) will give a strong index for retrieving by location:
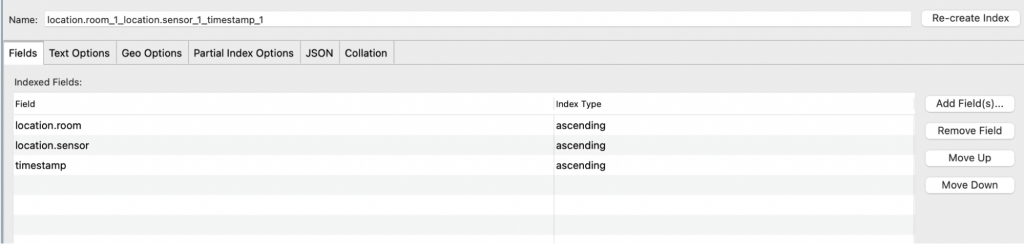
Aggregating with MongoDB Time Series Collections
The real power of Time Series comes when you need to aggregate the data. With the support for Time Series collections came a number of new aggregation stages built with this kind of data in mind. That doesn’t mean that these stages only work with Time Series collections, just that they work better with time series data. For example, we have written about the $setWindowFields stage in the Studio 3T Blog using normal collections. But it can enable some powerful time series aggregations.
$setWindowFields
The problem with aggregation is that, without stashing data in variables, it regards each document in isolation. That’s where the $setWindowFields
comes in as it combines some of aggregation’s $group
functionality with window functions.
{
"partitionBy" : { location:"$location", day: { $dayOfYear: "$timestamp" } },
"output" : {
"averaged" : {
"$avg" : "$temperature",
"window" : {
documents: [ "unbounded", "unbounded" ]
}
}
}
}
The “partitionBy” section splits and groups the data up by our meta data and, in this case by the day of the year. The “output” section then defines what output should be added to every document, based on their partition. So our first field is an “averaged” field for the temperature. This will be the average for that partition. The “window” section says use the entire partition to work out the average, “unbounded”. The result of this stage is that every document gets the average temperature for its partition added to it.
Add on a $match:
{
"$expr": { $lt: [ "$temperature", "$averaged" ] }
}
And now the result of the aggregation is every incident when a location had a lower than average temperature for that day.
You can change that window to work with moving windows, adjusting the boundaries of the average calculation too. Or compare documents relative to each other. And if you want more than an average calculation, there’s a host of window operators including accumulators, ranking, ordering, and gap-filling.
Of course, you can use any aggregation you want with a Time Series collection. We’re just mentioning $setWindowFields
as it’s almost custom-made for Time Series data analysis.
End of Time Series
This is just a quick introduction to Time Series collections. You should now understand why you would use them (to reduce storage needs and optimize for time) and that there are MongoDB aggregations that will let you leverage your ability to efficiently store Time Series data. From version 2022.9, Studio 3T will let you create Time Series collections. A final note though, there are limitations on MongoDB Time Series collections which you should note as they are implicit to how they are implemented.